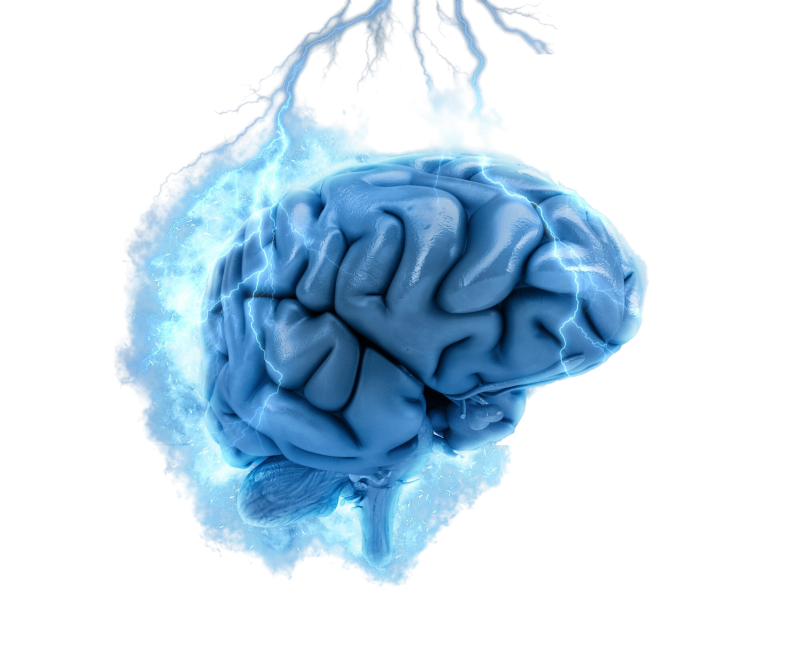
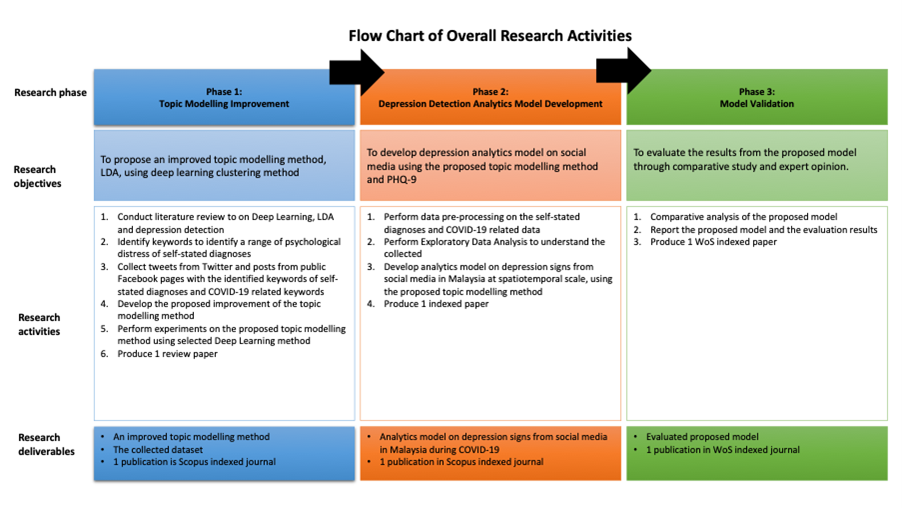
Due to the COVID-19 pandemic and the Malaysia government’s enforcement of Movement Control Order (MCO), majority of people in Malaysia have experienced a great deal of uncertainty about their social and economic stability across various sectors, and have increased people’s stress levels. Psychological studies using traditional surveys are time-consuming and contain both cognitive and sampling biases, and therefore cannot be used to build large datasets for a real-time depression analysis. Topic modelling technique, such as Latent Dirichlet Allocation (LDA) is one of the common approaches to analyse topics from given texts. However, LDA is based on unsupervised clustering method, thus it suffers from common clustering problems such as initializing of the number of clusters and cluster overlapping. The aim of this research is to analyse signs of depression from social media in Malaysia through an improved topic modelling technique via deep learning approaches, with Patient Health Questionnaire-9 at spatiotemporal scale in Malaysia during COVID-19 pandemic. Meanwhile, deep learning approaches have been shown to be potential in improving the performance of clustering methods. Hence, this research is going to be conducted in three phases, first is to propose an improved topic modelling method, LDA, using clustering method, second to develop depression analytics model on social media based on the proposed topic modelling method, and third, to evaluate the results of the proposed method through comparative study. It is expected that this research will produce a spatiotemporal analytical model of depression signs of Malaysia’s population through social media. The outcome of this research may contribute to other text-based research opportunities as it involved of producing Malaysia social media and mental health corpus. This research is in line with Malaysian agenda, the 10-10 MySTIE FRAMEWORK following Societal Well-Being Catalyst of Malaysia Socio-Economic Drivers under Medical and Healthcare, specifically Digital Health.
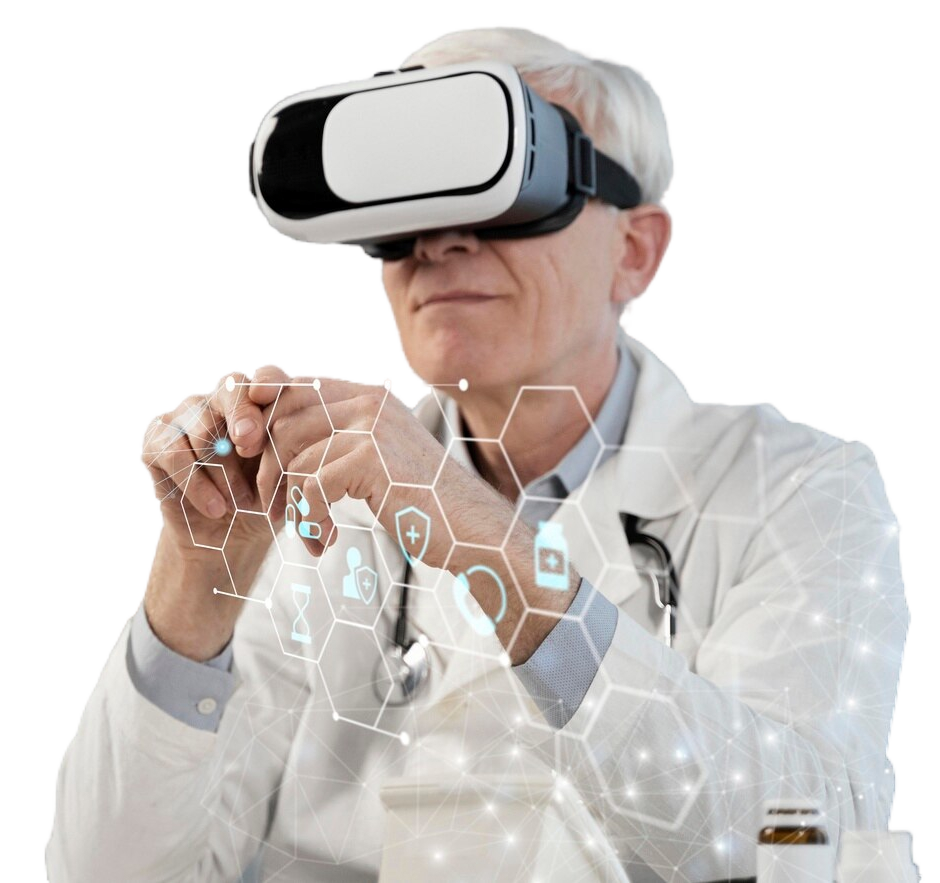
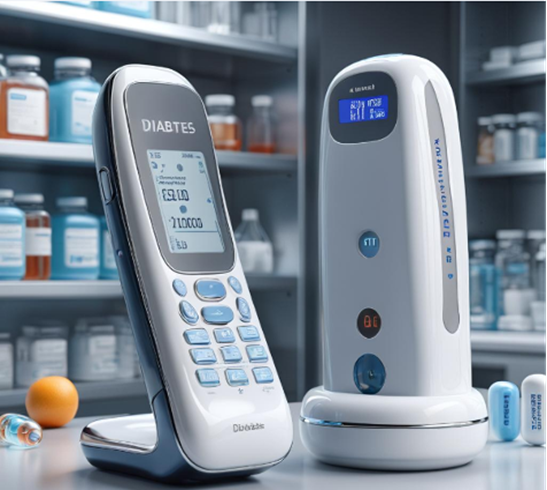
Compared with conventional diabetes management methods, artificial intelligence-based (AI-based) management offers significant advantages including low costs, easy implementation, broad coverage, flexible doctor-patient interaction, avoidance of repeated efforts, reduction in the workload of medical personnel, and an enhancement in effectiveness. Current AI technologies applied to diabetes management globally mainly focus on early diabetes education, diabetes prediction, lifestyle guidance, insulin injection guidance, blood sugar monitoring, self-management, and complication monitoring. There is a range of AI in healthcare and AI applications in diabetes management in the region. However, there is still no specific AI diabetic care tool for the Malaysian population. Thus, the Institute for Health Behavioural Research, Ministry of Health (MOH) Malaysia is currently working closely with the MySejahtera department to develop an AI: Diabetes Behavioural Diagnosis Instrument (DBDI) to provide Malaysians with an easy-to-use tool that allows people with diabetes to measure their current health behaviours and make positive changes in their health behaviours to achieve a healthier lifestyle and act as a systematic surveillance and evaluation system to monitor diabetes health behaviour risk factors that can help health practitioners. Therefore, to achieve the mission of MOH in developing an AI DBDI tool, it is essential to discover the patients’ attitudes toward this tool. A wide lens on the receptivity of AI among patients will be necessary to ensure that healthcare providers design services that are acceptable and trusted by all members of the community that these services are designed to serve. The AI technology is poised to revolutionize the modern delivery of healthcare services. Thus, this study aimed to explore the patients’ perspectives and attitudes towards AI tools for diabetic care.
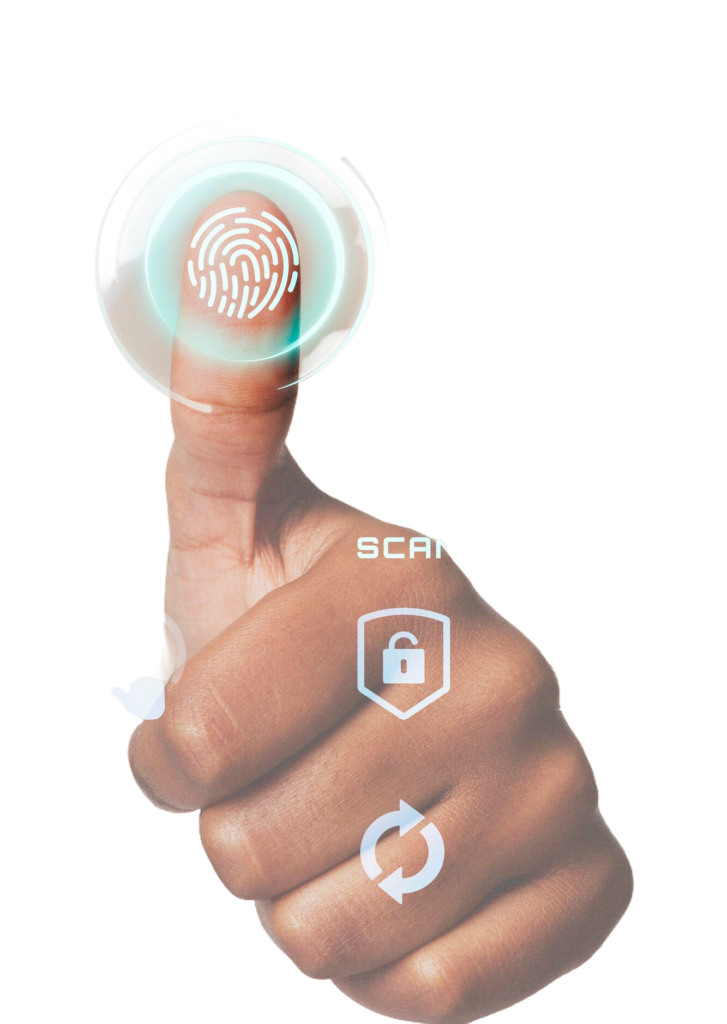
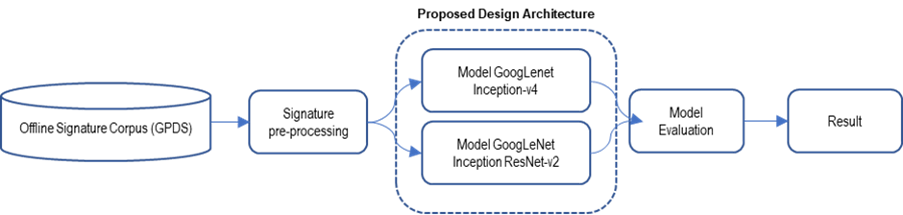
Handwritten signature remains relevant to the present year’s business necessity and is broadly used to tackle the forged signatures possibility; specifically, in banking, legal documents, and commercial transactions. Offline signature verification (OfSV) is essential in preventing the falsification of documents. Offline signature verification is a challenging task due to the fewer distinctive features possessed by static signature images. Deep learning (DL) based OfSVs require a high number of signature images to attain acceptable performance. A deep learning model such as a convolutional neural network is able to learn the visual cues directly from the static signature images automatically and obtain good features representation for verification purposes compared to the common machine learning process. It has a large number of model parameters that need to be trained to produce an acceptable result. The propose formulation of deep learning model effort could be reduced by using the data augmentation learning approach. Data augmentation learning employs the pre-trained model parameters to be used for a new model that deals with a similar problem such as vision tasks. Therefore, the purpose of this research is to propose and analyze of deep learning – data augmentation model based deep convolutional network models using GoogleNet Inception-v4 and Inception-ResNet-v2 asapproaches toward offline signature verification. The development of models is supported by the signature corpus collected from Grupo de Procesado Digital de Señales (GPDS), which comprised216,000 signatures from 4000 users with 24 genuine and 30 forged signatures for each user.
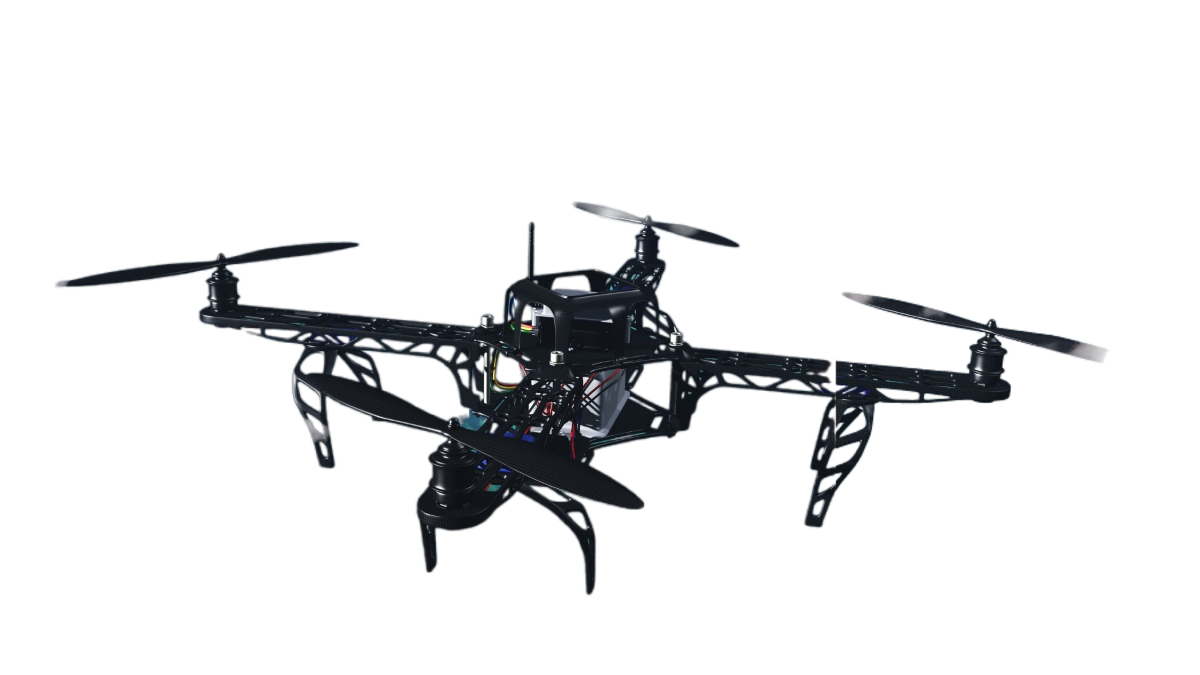
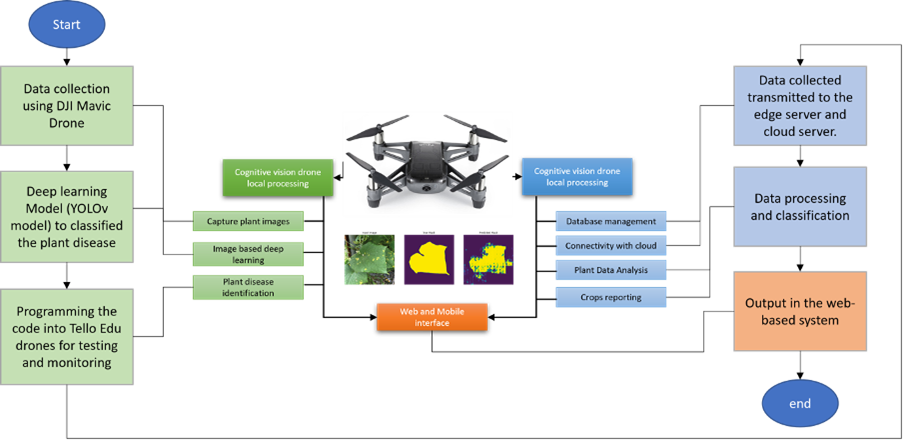
Integrating drone technology with plant disease detection is a significant advancement in agricultural surveillance, marking the beginning of a transformational era characterized by innovation. This study uses drone imagery in precision agriculture to explore the utility of You Only Look Once version 8 (YOLOv8), a powerful deep-learning model, for detecting diseases in melon leaves. Aerial data collection via drones yields high-resolution images, subsequently pre-processed for uniformity by resizing, removing irrelevant images, and extracting video frames. The labeled dataset is created by meticulously annotating disease-affected areas using bounding boxes. The YOLOv8 model has been trained using a labeled dataset to detect and classify various diseases accurately. When compared to other models, the YOLOv8 demonstrates exceptional performance. It has been shown that rigorous evaluation can help find diseases, which suggests that it could be used for early intervention in precision farming and to change how crop management systems work. This has the potential to assist farmers in promptly identifying and addressing plant issues, hence altering their crop management practices. Another part of the system is drone swarming. This system involved two Tello EDU drones for monitoring. Tello EDU drones are chosen for their popularity, which is attributed to their programmability, ease of use, and compact size. This system utilizes the Tello EDU drone because it is the only drone that can be programmed. The Tello EDU drones’ small size makes them practical in confined spaces like greenhouses, enabling the observation and monitoring of plants from various angles and heights. A pre-set path was followed by two drone swarms together before the experiment. The altitude for drone flight was determined based on the height of the plants. Currently, the system is offline, which means that footage is first captured by the drones, and subsequently, the recorded footage is processed using the YOLO model to analyze the condition of the melon plants. The system’s deployment begins with the drones swarming, during which the drones record the plants. Subsequently, after the recording is complete, the drone footage is saved on the computer as an .mp4 file. The computer then runs the deep learning models offline after the swarming phase. Following this process, the detection results can be analyzed once the deep learning model has been run on the recorded footage.
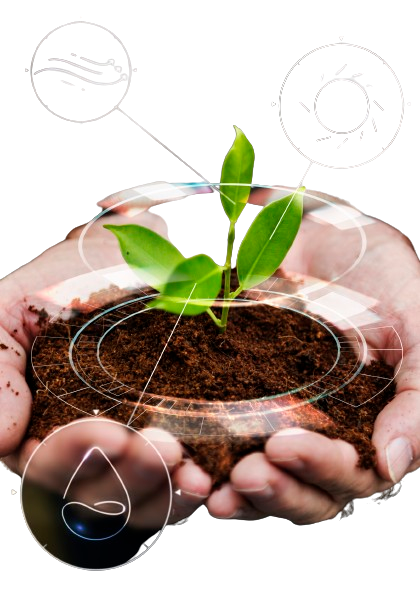
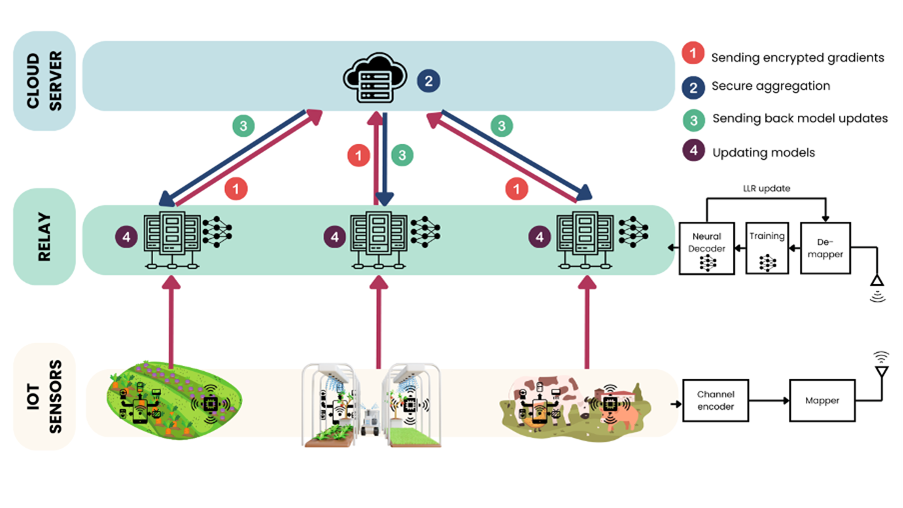
Modern agriculture applies information technology to improve the quality of agriculture based on efficient management and monitoring, called smart agriculture. However, the transmission of the wireless sensor networks will experience low-quality data in a large agricultural area. The application of some relay stations may help, but relay without careful algorithm design makes smart agriculture waste power and increase system complexity. Since the large area involves many sensors and relay stations, the link is also vulnerable to eavesdroppers stealing or disturbing the transmission of information. Therefore, a new channel coding based on Raptor-like coding with a fixed rate is proposed. The information is decodable based on deep learning and analysed to provide highly reliable results. We will investigate network security using FL and the frozen bits between relay and cloud links. A complete AGRO-FDL system performance evaluations are based on a series of computer simulations. The theoretical lower bound performance is derived mathematically using the outage performance analysis and related tools. This AGRO-FDL project expects to contribute a new channel coding scheme and its decoding to work optimally for disease prediction by exploiting the uniqueness of plant diseases pattern. The application of FL can guarantee a secured data transmission in the AGRO-FDL system. The output is significant to the agriculture sector to monitor large-scale plantation with an artificial intelligence (AI) system that can provide less power consumption and accurate prediction.
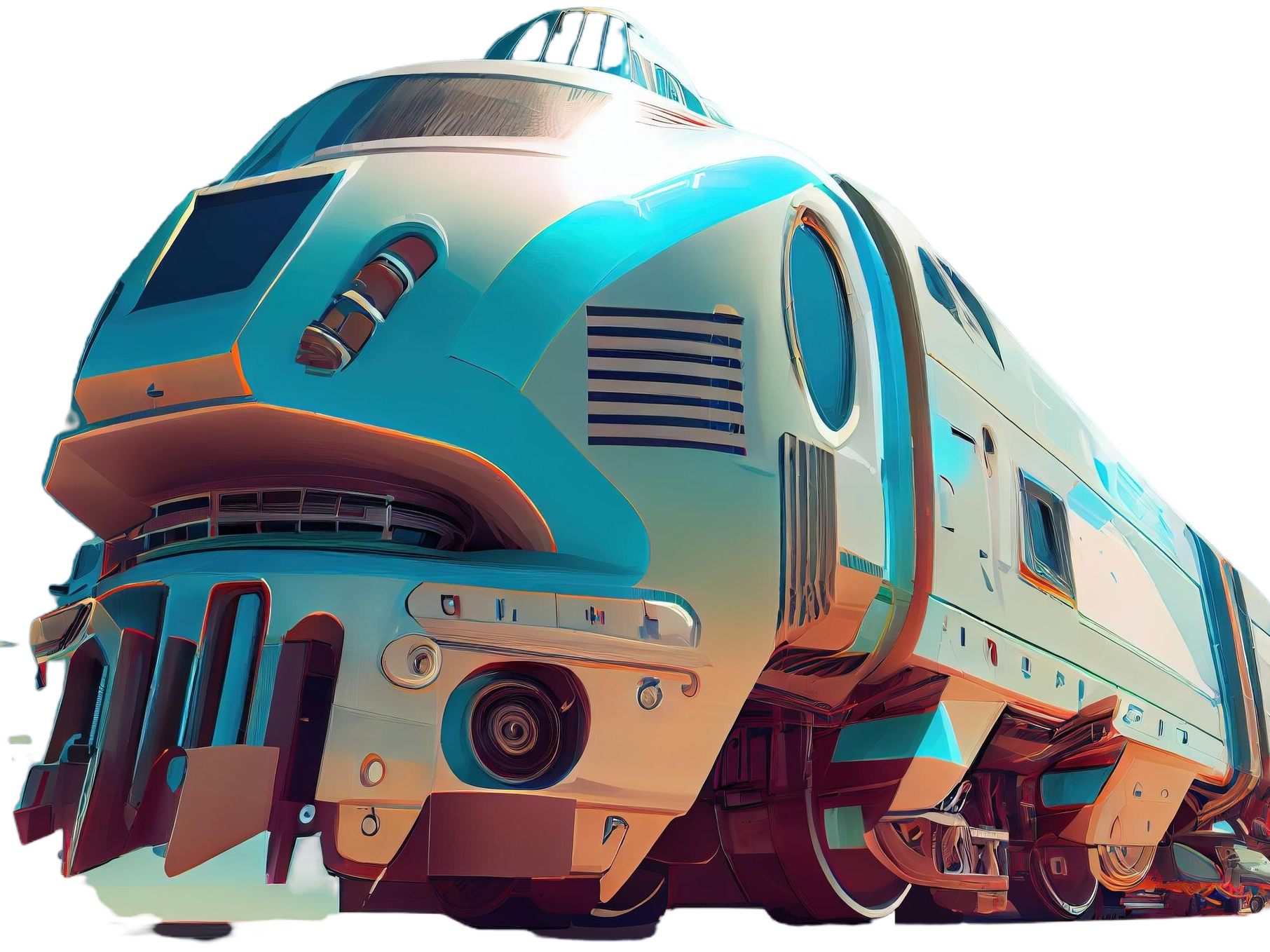
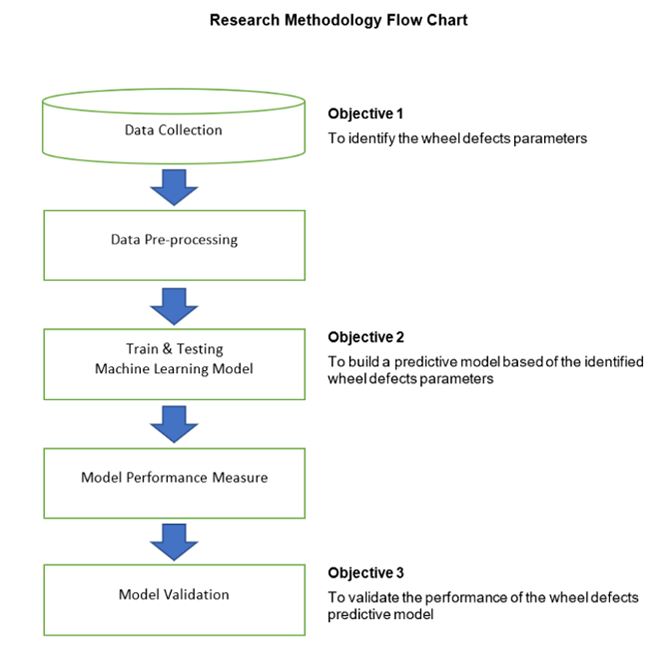
The rapid expansion of the railway network in both urban and rural areas is the proof that the population accepts rail transit. In order to ensure a safe and pleasurable ride, a train must be in outstanding condition. Thewheelset is among the most heavily worn components of the railroad. An abrupt change in train speed and frequent braking may cause the wheelset to degrade. Railway engineers still struggle to manually check forwheel defect, and poor operations planning will raise maintenance expenses. To determine the wheel conditions that are prone to failure, a railway wheel defect prediction must be carried out. Thus, the manual wheeldiagnostic process currently in use can be improved. The wheel defect types will be examined in this study using the wheel profiles data, collected from the MASS rapid transit trains. Using data on typical mechanicalsystem defects (cracks, fractures, and deformations), the periphery geometric shape of the successful wheel profiles will be compared to the unsuccessful wheel profiles. The wheel profile data will be taken fromthe underfloor wheel lathes machine, during the wheel re-profile. By using machine learning algorithm, a procedure to classify the wheel defect types can be materialized. Support vector machine for instance, a supervisedlearning approach will be used to identify the types of wheel defects. In terms of categorization of fault detection with different severity levels, the SVM model performed accurately and validly. Consequently, resulted awell-trained machine learning model which can predict the corresponding class to which a wheel defect belongs. This study is anticipated to extend the wheelset life, optimize the maintenance schedule and reducemaintenance costs, which also aligns with the SDG’s eleventh target – access to safe, affordable, accessible, and sustainable transportation systems, which highlighted the necessity to start this study.
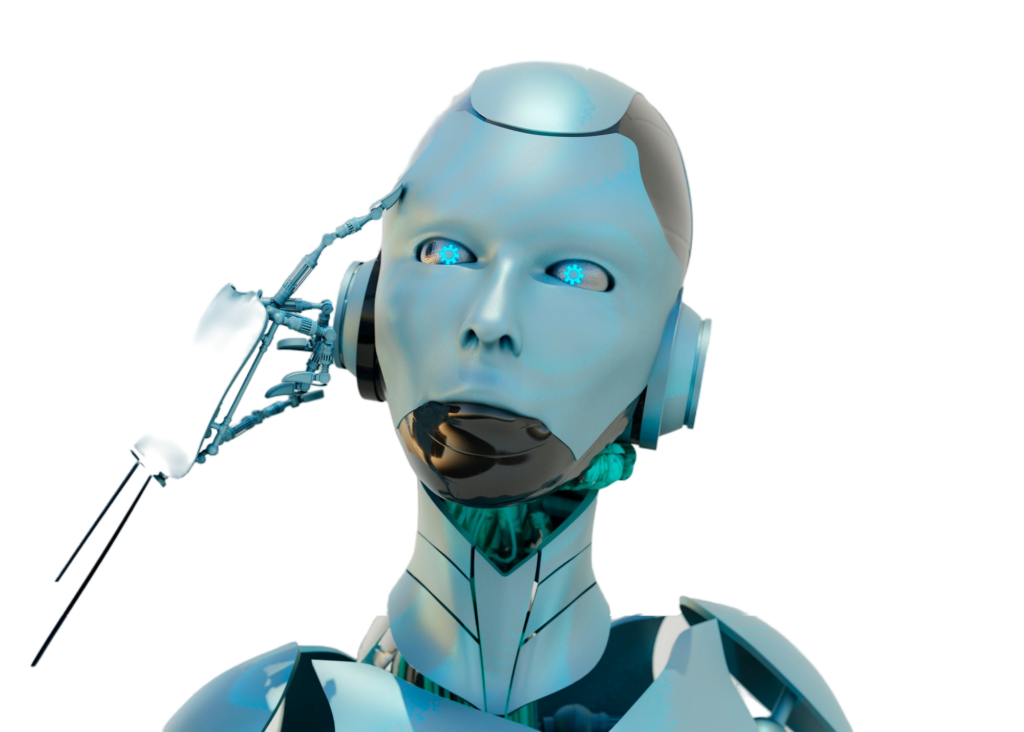
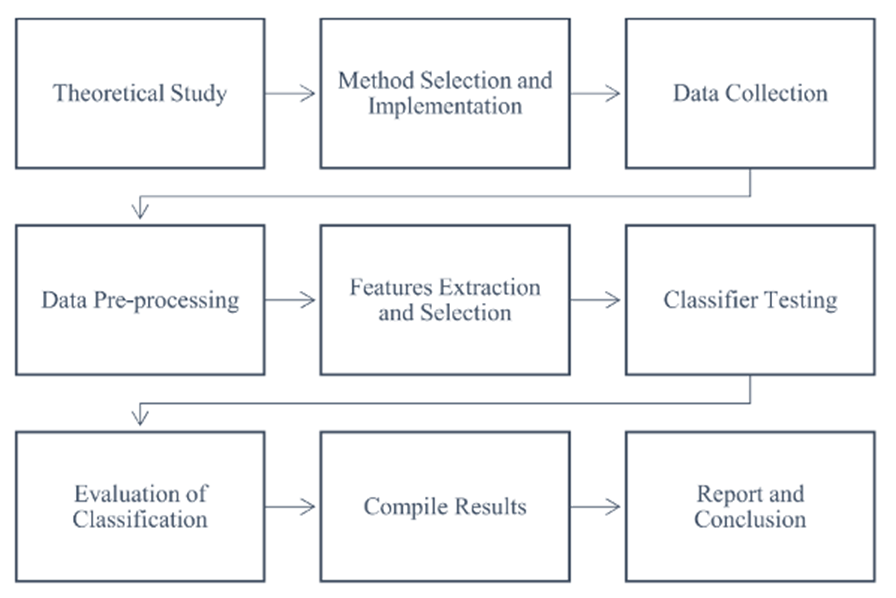
Depression has been affecting people all around the world, including Malaysians. Early detection mechanisms are vital for assisting clinical professionals in identifying depressed patients at an early stage. Although this can be accomplished through interviews and questionnaires, the time-consuming method has several additional disadvantages. Acoustic Measurement and MFCC have notably been adapted to detect speaker emotion. Numerous researchers have employed various languages for the purpose of prediction. Its efficiency varies across research, although it contributes significantly to diagnosing depression. As it appears that culture diversity influences how emotion is perceived, depression detection mechanism can vary between different languages. This project provides a comprehensive analysis based on relevant studies published from 2000 to 2023 to show the effectiveness of acoustic measurement and MFCC in depression detection. It was discovered that Support Vector Machine (SVM) is extensively utilised and can successfully contribute to the detection of depressed patients using biometric characteristics. The outcome of this project encourages experimental investigation on the effectiveness of acoustic measuring and MFCC for depression identification among Malaysian speakers.
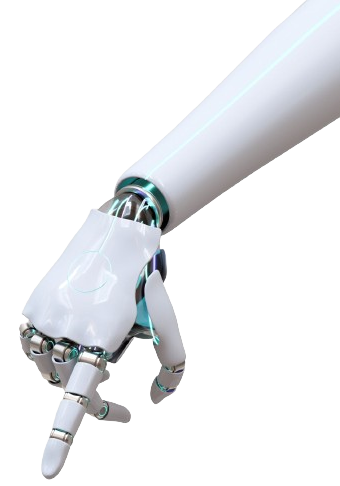
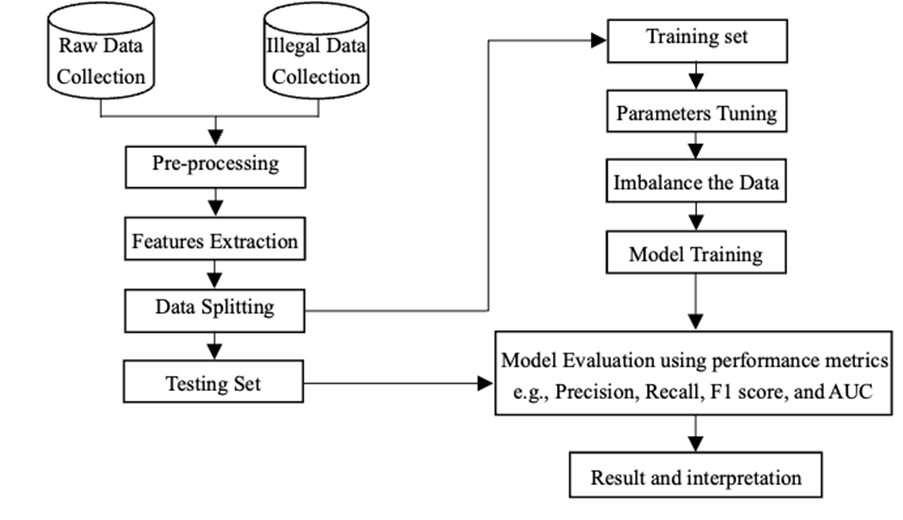
Shortly after its official launch in 2009, Bitcoin has gained rapid popularity worldwide, which in return attracted a variety of people especially malicious attackers, who get the advantage of its pseudo-anonymity to institute un-traceable threats, scams, and criminal activities. Recently, some Bitcoin thefts have been reported costing millions of dollars, causing serious harm and losses to innocent users or companies that lead them to declare bankruptcy. One of the main characteristics of Bitcoin is its anonymity, which makes Bitcoin the preferred choice for criminals to perform illicit activities that pose difficulties for law enforcement and financial authorities to identify suspicious behavior, making the existing fraud detection systems ineffective. In this project, we propose a model for detecting suspicious activities in the Bitcoin network. We first construct a labeled dataset by collecting a set of illicit transactions from public online Bitcoin forums, as well as datasets from prior research. Next, a verification and filtration process has been performed to verify the gathered illicit transactions with the original dataset and manually marked them as either legal or illegal. Additionally, a new set of features that are based on time-slice was extracted, the skewed dataset was balanced, and three supervised classifiers (LR, NB, and ANN) were used for evaluating the proposed model. Finally, our findings found that the ANN classifier achieved the best performer among others, which attained Precision, Recall, F1 scores, and AUC of 95.2%, 88.7%, 89.8%, and 91.2% respectively. The performance of the supervised classifiers has significantly improved after balancing the training set.
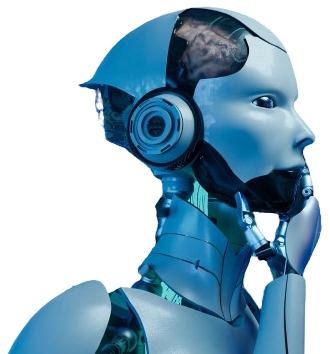
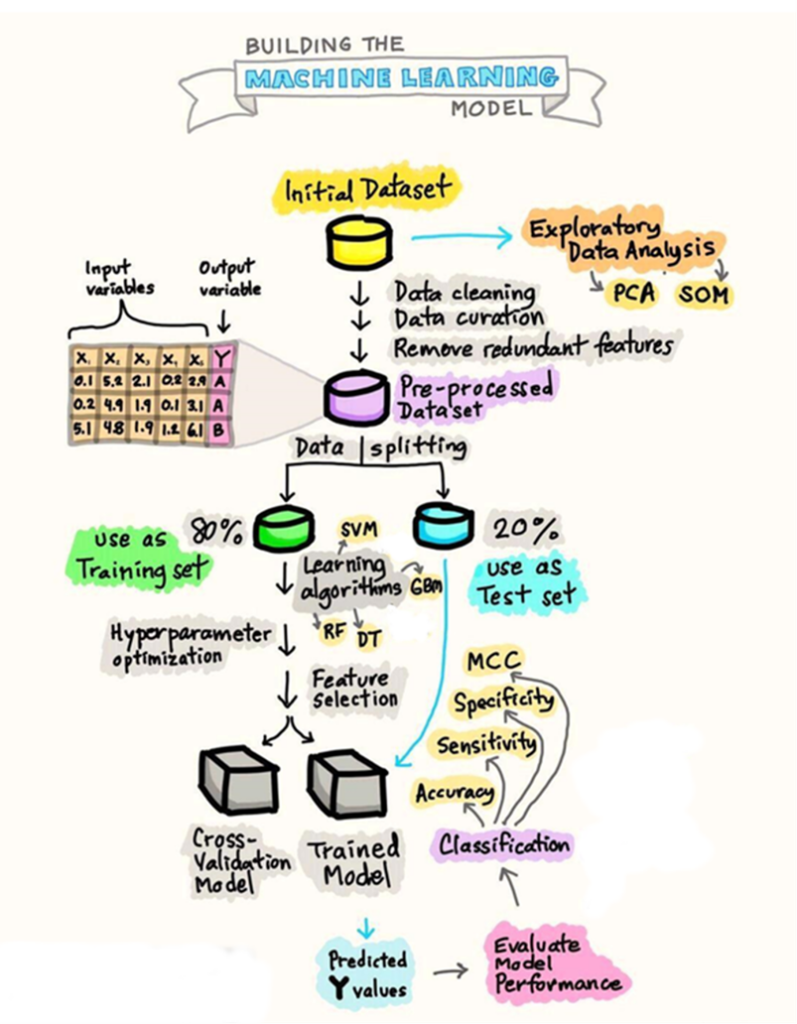
The world had undergone a life-changing impact of the Covid-19 pandemic, which began in Wuhan, China, in December 2019. The virus’s rapid global spread, leading the World Health Organization to declare it a pandemic by March 2020. Due to this situation, there is an urgent need to understand Covid-19 mortality risk factors. Machine learning can be a pivotal tool in this endeavour, aiding in the analysis and prediction of these risk factors, which help to focus on enhancing pandemic management strategies through targeted interventions and informed decision-making.
This project utilizes data collected directly from Sungai Buloh Hospital database, covering the period between August and September 2021, a peak time for Covid-19 cases and deaths in Malaysia. It includes 128 inpatients, equally divided between survivors and non-survivors. The dataset encompasses comprehensive data on sociodemographic characteristics, Covid-19 exposures, symptoms, health history, and laboratory test results. The study’s methodology involves machine learning techniques, employing advanced statistical models and data mining to identify significant risk factors associated with Covid-19 mortality. The idea is to provide an alternative to traditional statistical methodologies, identifying individuals at higher risk of mortality based on a range of demographic, clinical, and contextual factors.
The findings of this project indicate a predominance of the Malay race among Covid-19 inpatients at Sungai Buloh Hospital, with a wide age range among the patients. A majority had received at least one vaccine dose, and most cases were classified as severe. The study identifies 68 health-related features as relevant for mortality risk prediction, using five machine learning models: Logistic Regression, Decision Tree, Random Forest, Support Vector Machine, and XGBoost. The Support Vector Machine model showed the highest performance, with 94.9% accuracy, 95% precision, 95% recall, and a 95% F1-Score. Additionally, the SHAP model implementation with the Support Vector Machine identified 20 significant health-related features contributing to mortality risk prediction.
This project successfully achieved its objectives by developing a high-performing machine learning model for Covid-19 mortality risk prediction and identifying key health biomarkers related to increased mortality likelihood in Sungai Buloh Hospital’s inpatients. The Support Vector Machine outperformed other models in accuracy, precision, recall, and F1-Score. The SHAP model further elucidated significant biomarkers associated with developing mortality conditions, contributing to a deeper understanding of the complex interplay between various risk factors and Covid-19 outcomes. These insights are instrumental in enhancing pandemic management strategies.
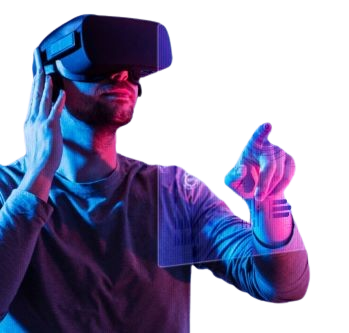
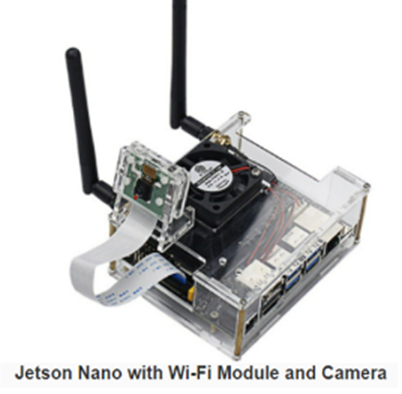
Health, security, and education constitute three main problems faced during the pandemic. The fast-spreading of the COVID-19 virus affects not only the physical health of the people but also their mental health, which reduces the performance of education and work of school kids and working adults, respectively. The temporarily abandoned schools, office buildings, and other properties also bring about security problems for the people besides affecting their emotions due to the prolonged stay at home. Even after the schools and commercial buildings reopen, detecting and monitoring the emotions and motions of the students and workers will remain a substantial issue to be addressed to support them in enhancing the quality of education, work, and life. The existing methods mostly target the creation of a smart network of intelligent agents or targeting certain types of buildings in the cities and not the health and emotion monitoring systems. A smart building model based on computer vision is proposed, focusing more on general object detection. Other methods are mostly designed for asset surveillance, object recognition, and virtually impaired person assistance. Therefore, a smart prototype to improve the health, security, and education quality levels is proposed in this project based on a discrete swarm intelligence and machine vision and detection system. Images in the target building will be captured via cameras before action and emotion detection are executed using the proposed smart intelligence system using Jetson Nano as the small processor to perform the detection. The objectives are to recognize the students’ and working adults’ actions and emotions and detect any suspicious movements. The outcome will be the prototype of the computer system based on Jetson Nano that will improve the health, security, and education quality levels not only during the pandemic but also after it is over. This aligns with Industrial Revolution 4.0, further accelerating the nation’s economy and education.
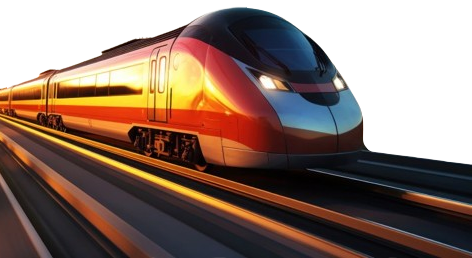
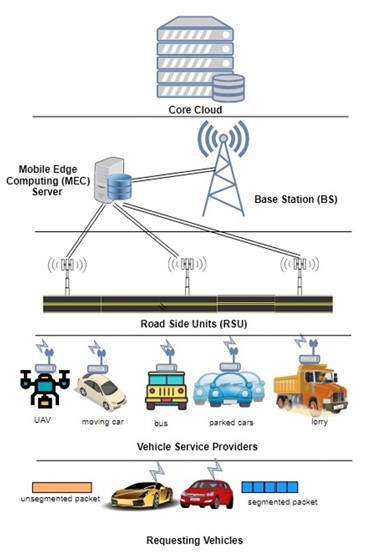
In the era of the intelligent transport system (ITS), autonomous vehicles will increase 10-100 times on the road relatively to today. Thus, the cloud computing platform will be congested and overloaded as autonomous vehicles are indeed associated with massive sensors and data for processing. The proximity of autonomous vehicles to cloud results in a latency problem that will interrupt the operation of the autonomous vehicle system, and this become severe for the case of road-safety services. Employing fog or edge nodes in the ITS can be a great solution as the data will be offloaded to a number of nodes for fast computation. Therefore, the key objective of this research is to design and formulate vehicular fog computing (VFC) called HOPING in the context of a resource management problem. Inspired by recent advances in machine learning, deep reinforcement learning based resource management (DRL-RM) is explored. In this study, we propose a joint optimisation by considering the cost, reward, and revenue of autonomous vehicles, vehicle edge node (VEN) and also a service provider, respectively while the latency is well-kept. The performance of DRL-RM is analysed and validated with previous algorithms via simulation work using MATLAB simulations. In addition to that, the impact of mobility of autonomous vehicles and VEN on the performance of HOPING is also examined. It is expected that the proposed DRL-RM can satisfy the required latency besides offering significant benefits to all parties involved. The significance of the study is that an advanced machine learning with the advantage of adaptive to the environmental variations is employed to solve the vital resource management issue of an autonomous vehicle, which greatly contributes to a national automotive industry.